Regression Analysis
Machine Learning
Regression is an family of algorithms used in machine learning where the main goal is prediction. It is often worth the time undertaking regression analysis as part of data exploration to understand the relationships between variables.
Regression analysis is suited to supervised learning tasks where one or more inputs are used to predict an output. Data can be linear and non-linear.
Regression can be used when a researcher has some understanding about the relationship between inputs and outputs. This can be based on theory or prior knowledge. The types of regression are:
- Linear
- Polynomial
- Generalised
- Ridge and lasso
- Splines
When the relationships between inputs and outputs is complex or unknown, a neural network can used used in place of regression.
Undertaking regression analysis as a machine learning exercise allows for the use of larger datasets and iterative training.
Econometrics
Regression is also a key tool in econometric analysis, inference and hypothesis testing.
The emphasis is on testing economic theories and understanding the significance of relationships between variables.
A range of criteria are used to determine whether the model has statistical significance and supports the theory under consideration.
Models are normally estimated using a closed-form calculation using ordinary least squares. This is in contrast to a machine learning approach that normally uses gradient descent.
Use cases
We can assist your organisation in determining whether regression analysis is a suitable tool for modeling and undertaking inference on your data and which approach is best based on your requirements.
Typical regression projects
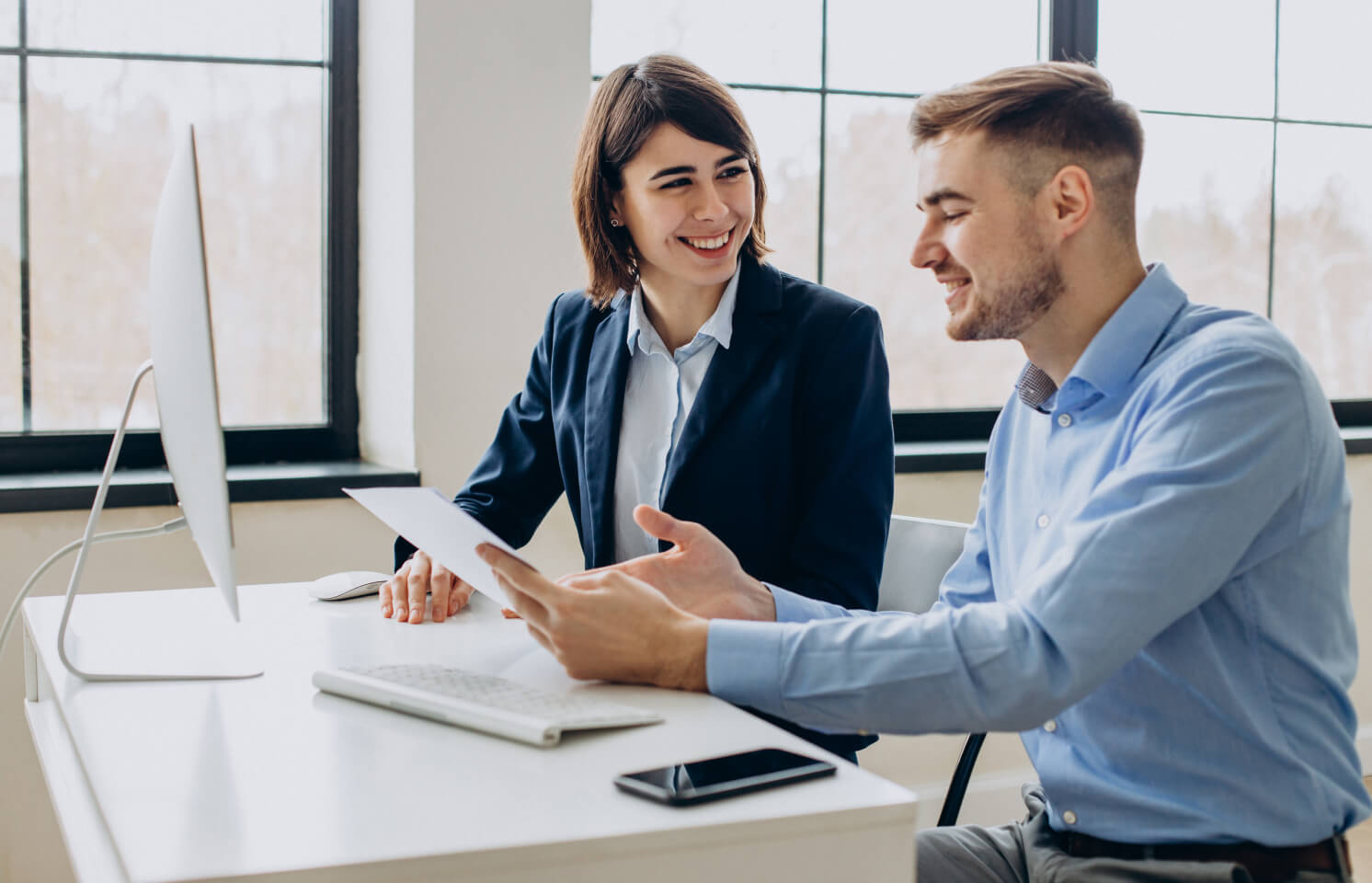
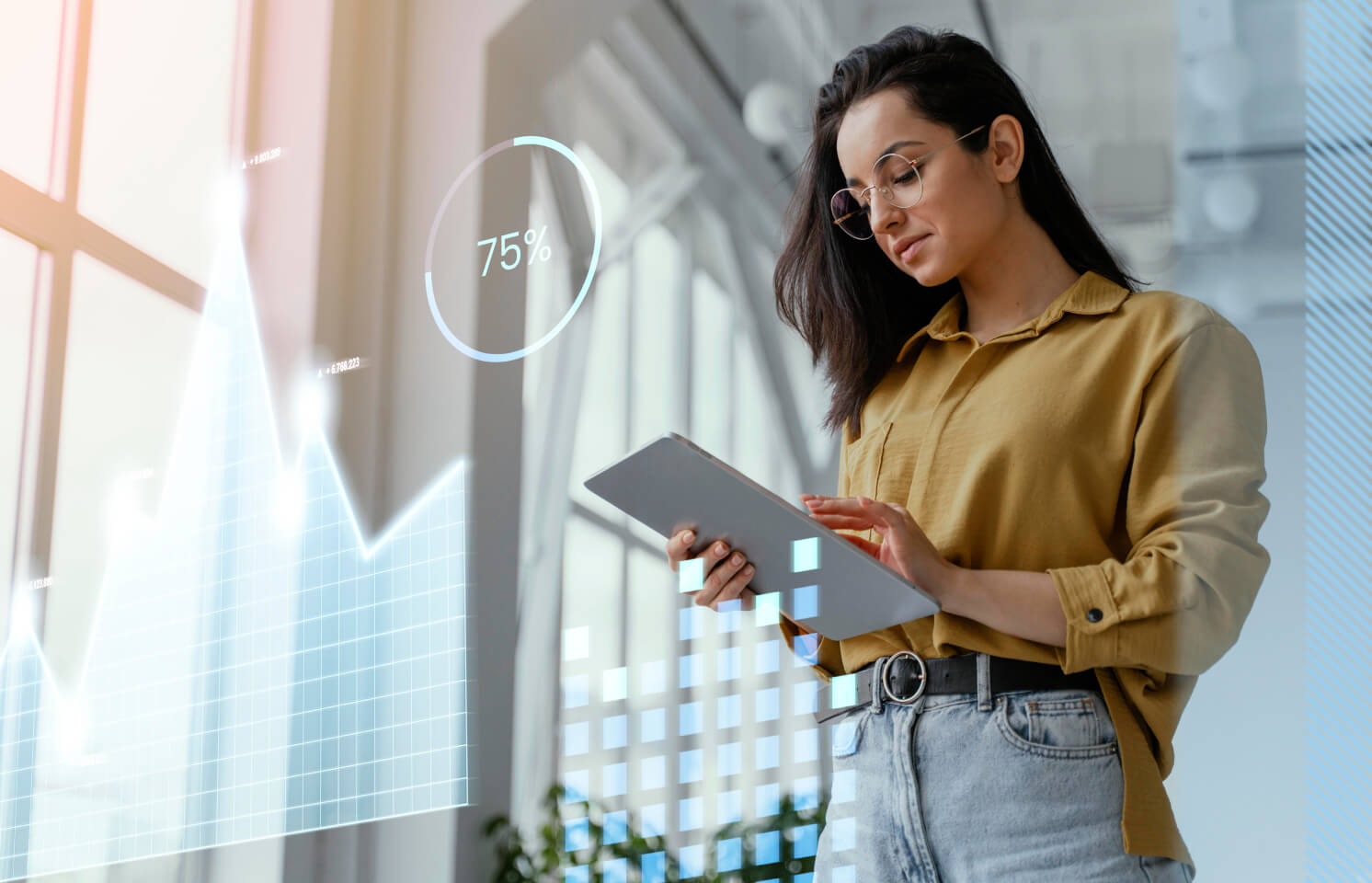
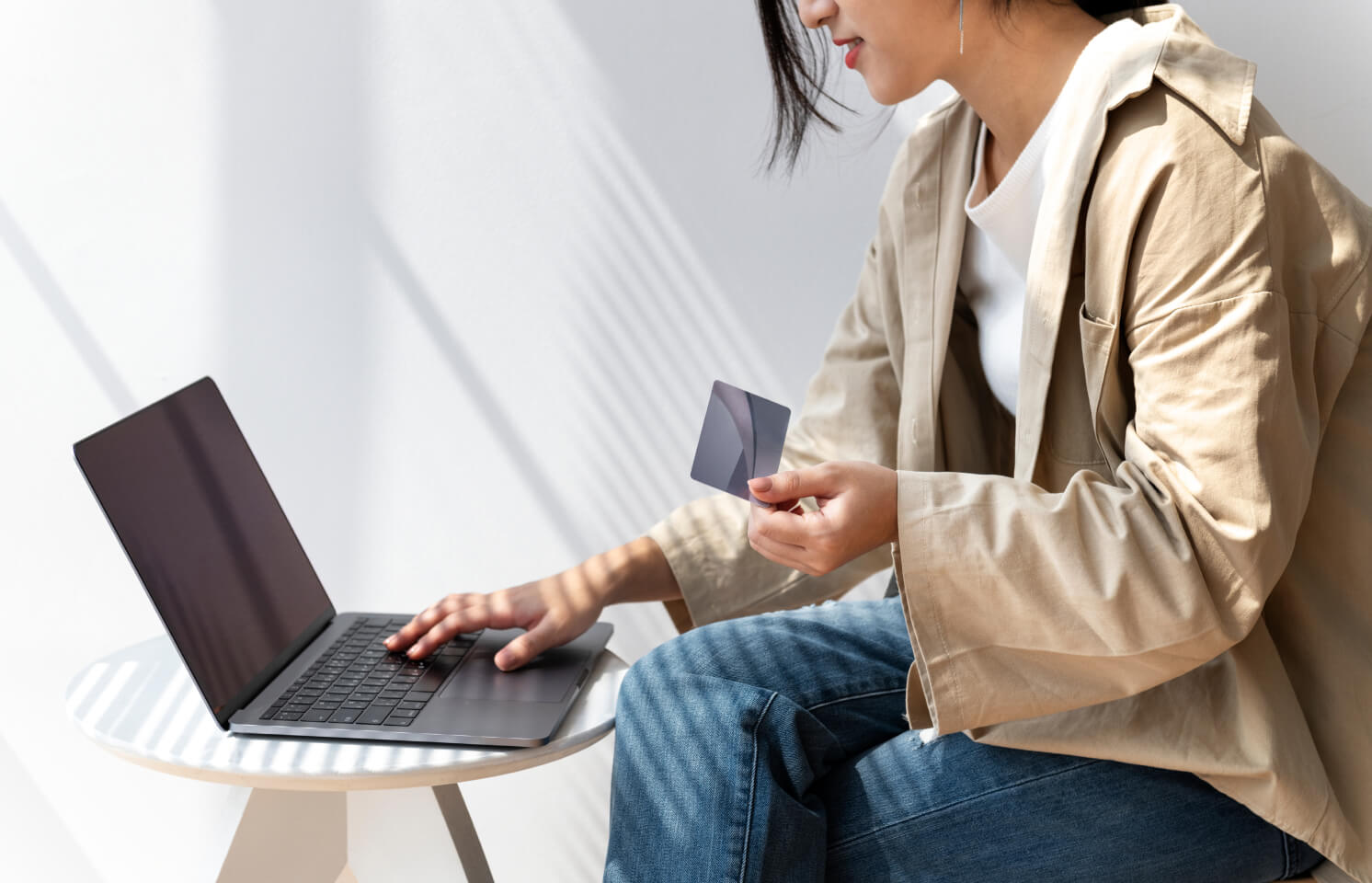
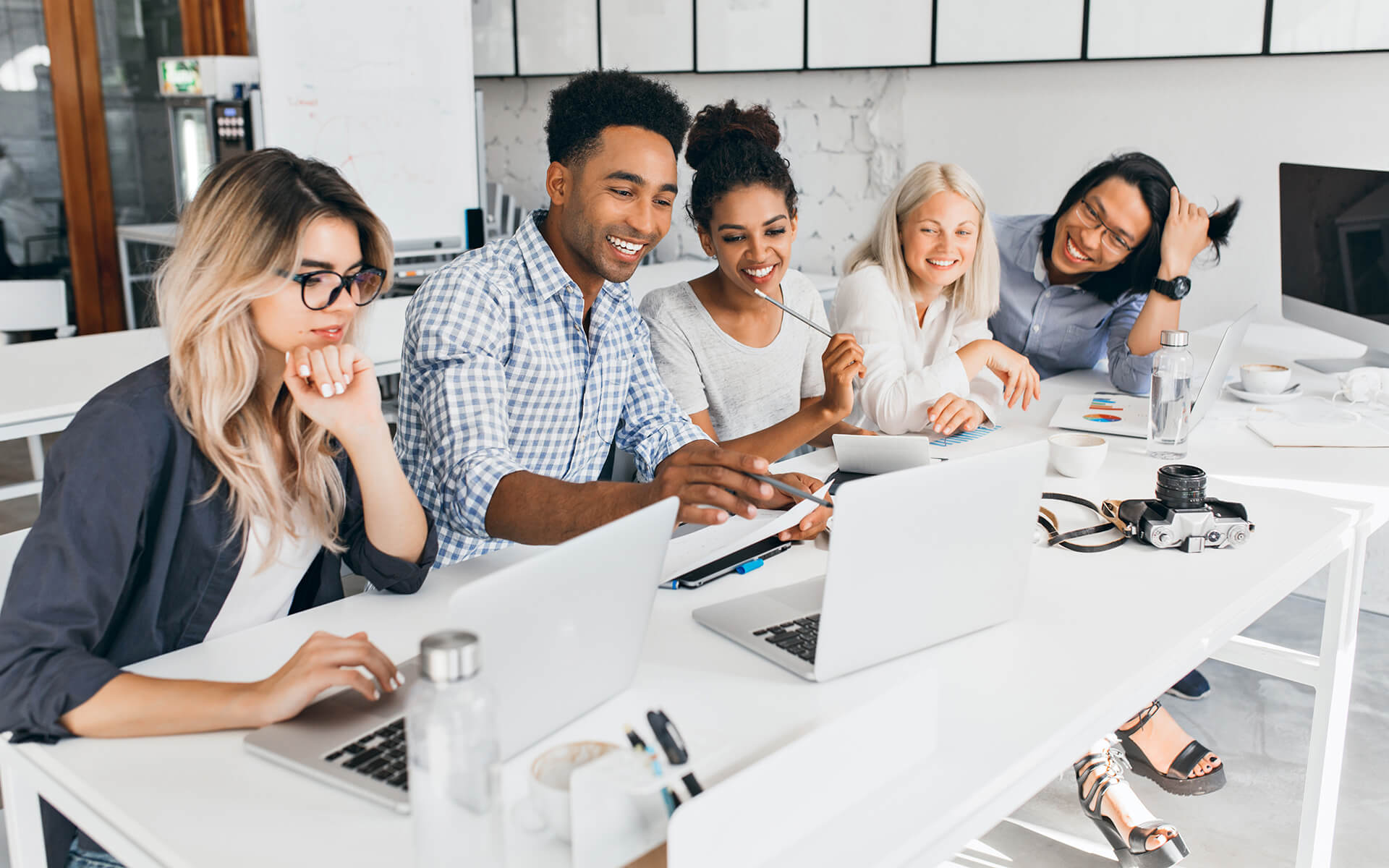